Vol. 48 No. 3, 2009
Identifying Biodiversity Hotspots by Predictive Models: A Case Study Using Taiwan’s Endemic Bird Species
Chia-Ying Ko1, Ruey-Shing Lin2, Tzung-Su Ding3, Chih-Hao Hsieh4, and Pei-Fen Lee1,5,*
1Institute of Ecology and Evolutionary Biology, National Taiwan University, Taipei 106, Taiwan
2Endemic Species Research Institute, 1 Ming-Shen East Road, Jiji, Nantou 552, Taiwan
3School of Forestry and Resource Conservation, National Taiwan University, Taipei 106, Taiwan
4Institute of Oceanography, National Taiwan University, Taipei 106, Taiwan
5Department of Life Science, National Taiwan University, Taipei 106, Taiwan
Chia-Ying Ko, Ruey-Shing Lin, Tzung-Su Ding, Chih-Hao Hsieh, and Pei-Fen Lee (2009)
Predicting species distributions and identifying biodiversity hotspots
are essential in designing conservation strategies. Because of
different spatial scales and/or species characteristics, uncertainty
still exist as to which model is the best. Several models have
been proposed to calculate the probability of species occurrences,
predict biodiversity hotspots, and decide importance levels of those
hotspots. We constructed predictive distribution models for 14 of
16 endemic bird species in Taiwan using a fineresolution (1 × 1 km)
breeding bird distribution dataset compiled over the past decade as
well as environmental variables. We compared the performances of
the 4 models: logistic regression (LR), multiple discriminant analysis
(MDA), genetic algorithm for rule-set prediction (GARP), and artificial
neural network (ANN). Maps for biodiversity hotspots were
generated based on the species distributions from the 4 models.
To account for potential uncertainty, we constructed hotspot maps using
a frequency histogram and probability density function
approaches. Based on the distribution maps and the area under the
curve (AUC) of the receiver operating characteristic, all of our models
made good predictions for each species (all AUC values were >
0.75). The nonlinear models (GARP, ANN, and LR) provided better
predictions than did the linear (MDA) model. GARP was the most
consistent model when evaluated by it kappa, sensitivity, accuracy, and
specificity values for each species and the 3 species categories
(common, uncommon, and rare species). The prevalence of all
species did not affect the final predictive performance. The 5
biodiversity hotspot maps derived from the frequency histogram approach
showed a relatively similar pattern to maps generated by the
probability density function, which indicated that of mid- to
high-elevation areas had higher probabilities. In spite of some
inconsistencies, the hotspot maps identified from these 2 approaches
were fairly representative when evaluated against currently known
hotspots. A GAP analysis indicated only 25% of the hotspots are
currently protected by national parks. We concluded that the LR,
GARP, ANN, and MDA models are all feasible to use for modeling bird
species distributions. Although there were some limitations, we
suggest using a combination approach to identify common features and
conservation priorities of biodiversity hotspots. Comparing known
and predicted hotspots can promote the reliability of the models as
well as provide managers with greater confidence when planning
conservation policies. Finally, this approach to identifying
common features and conservation priorities of biodiversity hotspots
can be applied to evaluate conservation efforts and provide a better
tool to achieve efficient conservation.
Key words: Conservation, Biogeography, Endemism, Model, Biodiversity.
*Correspondence: Tel: 886-2-33662469. Fax: 886-2-23623750. E-mail:leepf@ntu.edu.tw
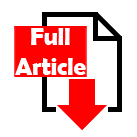
|